A Product Manager’s Guide to bringing out the "Good" in AI Models
As AI adoption accelerates, one question comes up repeatedly: What makes an AI model truly good? As a Product Manager advising teams on Google Cloud’s Vertex AI and other platforms, my role is to define, measure, and operationalize "goodness". A step to move from MLOps to BehaviorOps.
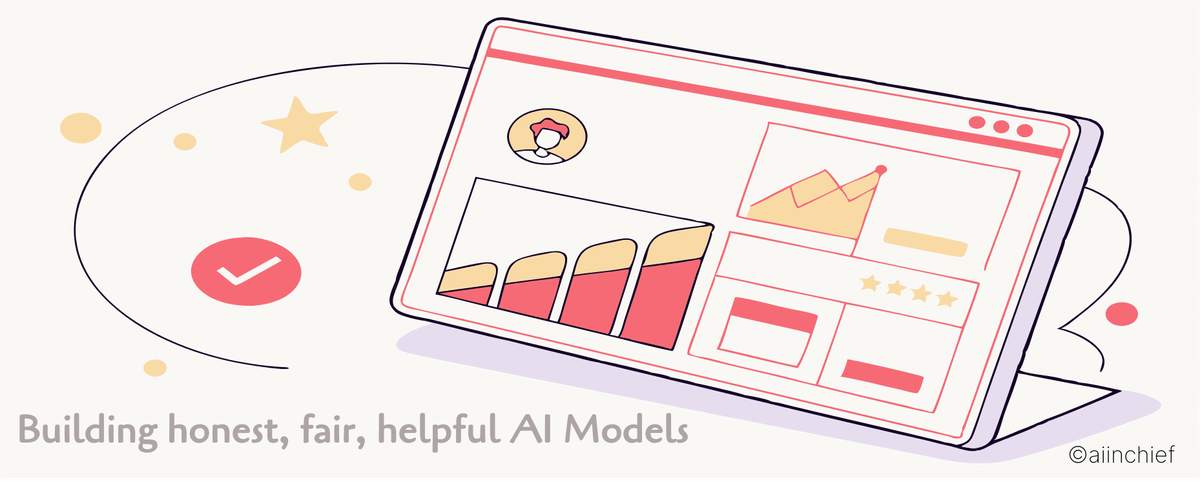
As AI adoption accelerates, one question comes up repeatedly: What makes an AI model truly good? As a Product Manager advising teams on Google Cloud’s Vertex AI and other platforms, my role is to define, measure, and operationalize "goodness"—not just as model accuracy, but as a full-stack behavioral framework.
This guide is your step-by-step playbook to building responsible, helpful, and fair models on GCP. A step to move from MLOps to BehaviorOps
🌟 Step 1: Define "Goodness" in AI — Beyond Accuracy
A good model isn’t just accurate—it’s aligned with human values and safe in its outputs. Here’s a working definition of model behavior:
“A good model is helpful, honest, and fair, delivering accurate outputs while maintaining trust, transparency, and inclusivity.”
We break this down into three categories:
- Functional: Accuracy, latency, throughput.
- Behavioral: Helpfulness, honesty, fairness, adaptability.
- Operational: Reproducibility, transparency, safety.
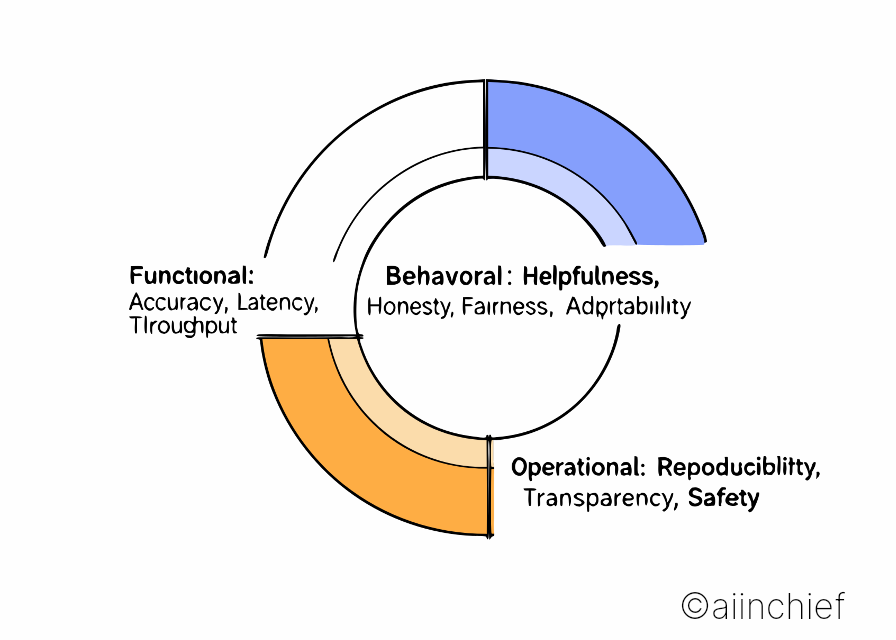
🗺️ Step 2: Map the Metrics — Performance vs. Behavior
Let’s compare how traditional and modern model evaluations stack up:
Dimension | Behavior-Centric Metric | GCP Vertex AI Support |
Accuracy | Outcome alignment | AutoML, Vertex Pipelines |
Latency | Responsiveness under load | Vertex Endpoints, Auto-scaling |
Explainability | SHAP, LIME, Model Cards | Explainable AI (Vertex AI) |
Fairness | Demographic parity, equal opportunity | What-If Tool, Fairness Indicators (TFX) |
Safety | Toxicity thresholds, harmful output checks | Content moderation integrations |
Honesty | Admitting uncertainty | Multi-model fallback / prompt design |
Reproducibility | Full lineage and metadata | Pipeline Metadata Store, Model Registry |
The shift is clear: From evaluating raw performance to evaluating alignment with human needs.
♻️ Step 3: Implement the Lifecycle Using Vertex AI
To ensure these dimensions are built in—not bolted on—we follow a full-lifecycle MLOps setup using Vertex AI:
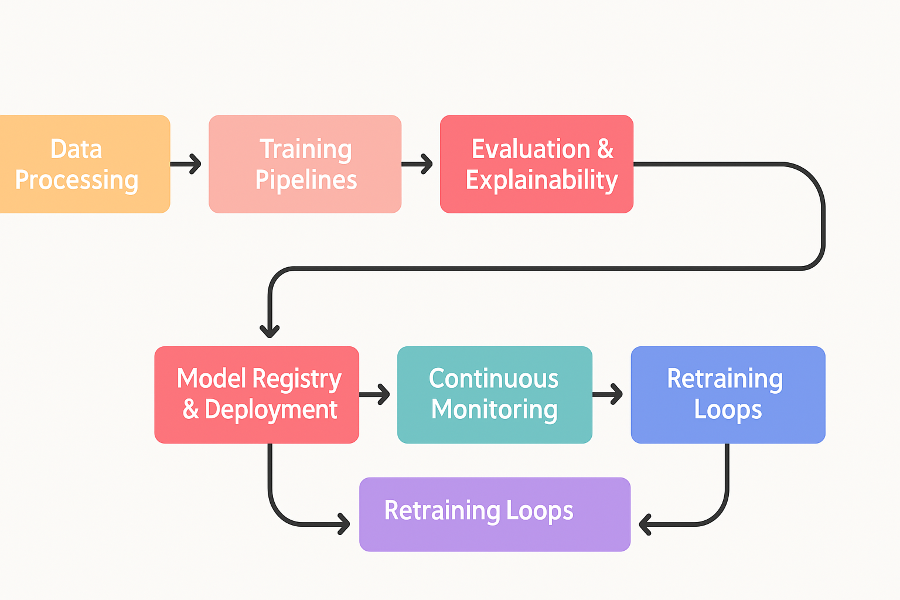
- Data Processing: Use BigQuery + Dataflow for ETL pipelines. Integrate Data Validation.
- Training Pipelines: Automate with Vertex Pipelines. Use Hyperparameter tuning.
- Evaluation & Explainability: Integrate SHAP via Explainable AI APIs.
- Bias & Fairness: Use TFX’s What-If Tool and Fairness Indicators for auditing.
- Model Registry & Deployment: Register validated models, deploy via endpoints.
- Continuous Monitoring: Track model drift and serving anomalies. Alert on data or concept drift.
- Retraining Loops: Trigger Vertex Pipelines based on drift events.
🎯 Product Tip: Bake your model behavior guardrails into every phase—don’t wait till deployment.
🧠 Step 4: Product Guidance for Each Key Behavior
Behavior | What It Looks Like | Vertex AI Implementation |
Helpfulness | Answers the actual user intent | Prompt tuning, user feedback loops |
Honesty | Says "I don't know" when unsure | Multi-model fallback or intent routing |
Fairness | Treats all users equitably | Fairness Indicators + What-If analysis |
Safety | Avoids toxicity, misinformation | Moderation classifiers, prompt filters |
Transparency | Explains why it made a prediction | SHAP, Model Cards, Logging |
Reproducibility | Consistent outputs across runs | Metadata tracking + CI/CD integration |
🏭 Real-World Example: AI in Manufacturing Quality Control
A global manufacturing company implemented a predictive quality model using Vertex AI to reduce defect rates on their production line. Initially, the model delivered over 93% accuracy but flagged high false positives for specific machine lines.
The problem? The model was trained on over-represented data from one shift and failed to generalize fairly across other lines.
The solution?
- Using Vertex AI’s Fairness Indicators, the team uncovered demographic skew by shift and location.
- Implemented SHAP-based explainability to communicate why the model flagged certain items.
- Incorporated continuous monitoring to identify drift across batches.
The result? Reduced false positives by 41%, improved operator trust, and avoided unnecessary downtime worth $200K/month.
This wasn’t just about model performance—it was about model behavior that earns trust at scale.
🚀 Final Word: From MLOps to BehaviorOps
We’ve evolved from building models to building behavioral systems.
As a Product Manager guiding AI initiatives, your job isn’t just to ship models—it’s to ship models that behave well.
With GCP Vertex AI, we can encode our product philosophy into real-world systems:
- Structure for reproducibility
- Metrics for human alignment
- Guardrails for safety and fairness
Let’s redefine what it means to say an AI model is “good.”
⚙️Open-Source Options for Lean Teams
Not every org needs full Vertex AI. You can build similar pipelines using open-source components:
Vertex AI Capability | Open-Source Alternative |
Pipelines & Metadata | Kubeflow Pipelines + MLMD |
Explainability | SHAP, LIME |
Fairness Auditing | Aequitas, IBM AI Fairness 360 |
Serving & Monitoring | MLflow + Prometheus/Grafana |
CI/CD | GitHub Actions + KServe + Argo Workflows |
Let’s Discuss 👇 What’s one trait you think every responsible AI model must have? Tag your favorite AI PMs and let’s debate what “good AI” truly means.