LLM Architect's Career Bridge for Future in AI
Large Language Models (LLMs) are shaping the future. At the center of this transformation is a powerful new role: the LLM Architect. If you're passionate about combining language intelligence with robust systems thinking- this career is your gateway to the future of AI.
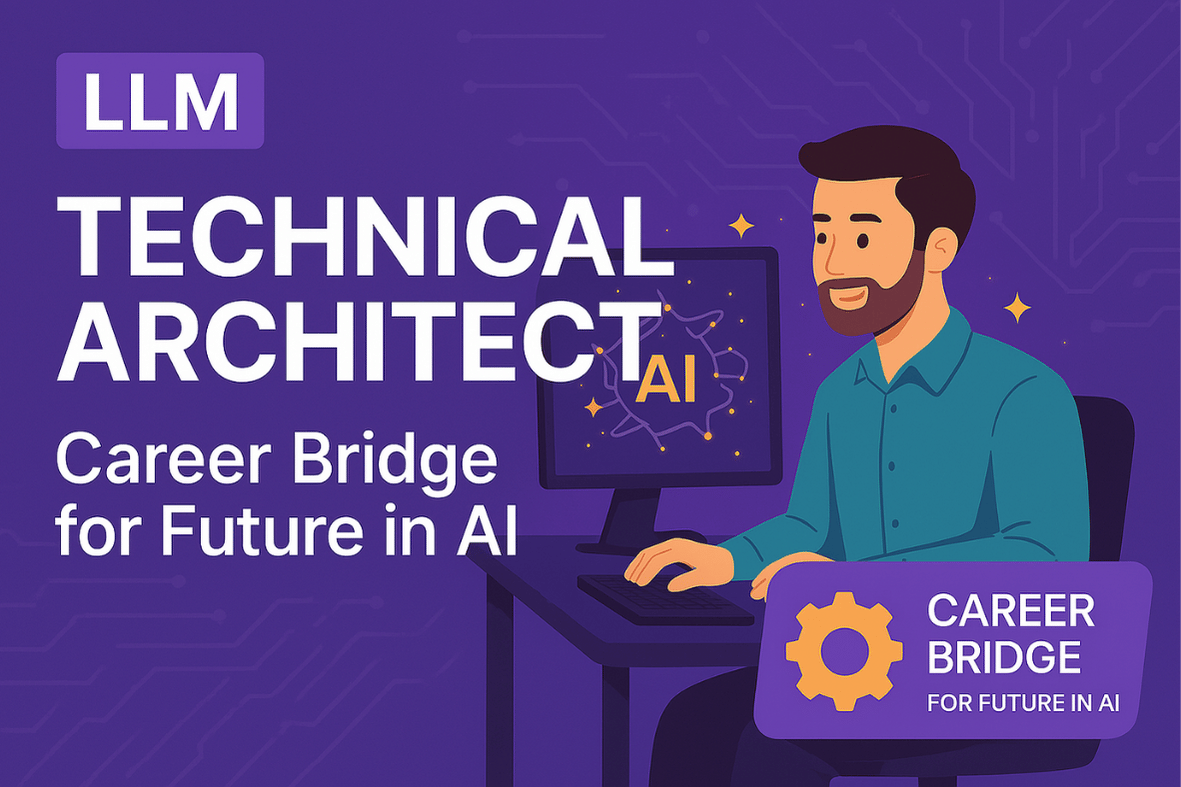
🧠A Successful LLM Architect
An LLM Architect is a visionary system designer who understands how to harness LLMs and integrate them seamlessly into scalable, secure, and intelligent applications. Unlike a data scientist or ML engineer, the LLM Architect is responsible for system-wide decisions that influence model performance, deployment, integration, and user experience.
They bridge the gap between AI potential and real-world implementation.
📘 What You Will Learn
In this article, you’ll uncover:
- The core definition and value of an LLM Architect
- Why this role is crucial in future-proofing businesses
- The tools, techniques, and mindsets to master
- How to present your skills for LLM Architect roles
- A time-based learning roadmap to break into this space
🚀 Why This Role Matters in the AI Future
The surge of LLMs in industries - from legal to healthcare to finance - demands architect-level leadership that goes beyond model training. LLMs are powerful, but without thoughtful system design, they risk being:
- Too costly to scale
- Too biased to trust
- Too complex to manage
LLM Architects bring clarity, structure, and innovation to LLM-powered solutions by defining how models interact with data, tools, users, and the business.
✅ Key Success Factors for LLM Architects
- Strategic Design Thinking: Seeing the LLM as a component in a larger business workflow
- Deep Technical Insight: Understanding model behavior, token usage, embedding, and optimization
- Modular System Architecture: Designing reusable, scalable components (prompt pipelines, caching, orchestration layers)
- Safety & Compliance: Building guardrails, moderation systems, and ethical filters
- Performance Optimization: Reducing latency and cost while maintaining accuracy
🧩 Key Responsibilities of an LLM Architect
- Design the end-to-end architecture of LLM-based products and platforms
- Evaluate and select appropriate LLM APIs, open-source models, or hybrid solutions
- Lead implementation of RAG pipelines, memory systems, and prompt strategies
- Define SLAs, performance metrics, and evaluation frameworks
- Collaborate with AI/ML, DevOps, and product teams for deployment and scaling
- Ensure systems comply with data privacy, security, and ethical standards
🧠 Core Capabilities Required
Technical Skills:
- Advanced knowledge of LLM APIs (OpenAI, Anthropic, HuggingFace, etc.)
- Hands-on experience with retrieval, embedding, prompt engineering, and tool integrations
- Experience with multi-turn conversations, context window management, and agentic behavior
System & Infra Knowledge:
- Cloud-native development (GCP, AWS, Azure)
- Containerization, serverless deployments, and streaming architectures
- CI/CD for ML workflows and AI observability
Soft & Strategic Capabilities:
- Stakeholder communication
- AI ethics and governance understanding
- Cross-functional leadership and project planning
🛠️ Top Tools to Learn for Interviews & Projects
Domain | Tools |
---|---|
LLM APIs & Platforms | OpenAI, Claude, HuggingFace Transformers, Cohere |
Prompt Engineering | LangChain, PromptLayer, Guidance, Promptfoo |
RAG & Vector DBs | LlamaIndex, ChromaDB, Pinecone, FAISS, Weaviate |
Evaluation & Testing | Ragas, TruLens, LangSmith |
Deployment & Monitoring | FastAPI, Streamlit, Docker, AgentOps, Langfuse |
📝 Top Resume Keywords for an LLM Architect
Make your profile stand out with keywords like:
- “LLM orchestration and system design”
- “RAG pipeline architecture”
- “Prompt templating and optimization”
- “Secure and scalable LLM deployment”
- “Vector search and semantic retrieval”
- “Content filtering and AI governance”
- “Cross-functional leadership for Gen AI systems”
📣 Don’t Have Experience Yet?
✨ No worries - start with us.
Join our hands-on LLM Architecture Internship Program where you’ll build real-world AI systems, guided by mentors who’ve deployed LLMs in production.
You’ll work on:
- System design challenges
- RAG implementation
- Prompt and function calling
- Performance analysis
- Project documentation for your resume and LinkedIn
⏱️ If You Only Had 1 Hour to Prepare…
Focus on the essentials:
- Read about RAG (Retrieval-Augmented Generation) - how it enhances model accuracy
- Understand prompt engineering basics: few-shot, zero-shot, temperature
- Explore OpenAI function calling & tools
- Map a simple use case: “LLM for internal knowledge assistant”
- Prepare 1 strong story of how you would design and improve it
📆 Prep Like a Pro: Your Learning Timeline
🕐 1 Day Plan
- Read a complete architecture case study from OpenAI or LangChain blog
- Build a basic LangChain app querying a vector store
- Update your resume and LinkedIn with LLM keywords
🧠 1 Week Plan
- Implement a basic RAG system using OpenAI and Chroma
- Build prompt templates for multiple tasks
- Monitor results using LangSmith or PromptLayer
🚀 4 Weeks Mastery
- Build a full-stack Gen AI application (UI + LLM + RAG + monitoring)
- Write a blog on Medium or LinkedIn about your learning experience
- Conduct mock interviews and share your portfolio
🔚 Final Thoughts
As AI adoption grows, the LLM Architect will become one of the most critical and in-demand tech roles globally. It's not just about knowing models - it's about knowing how to build real systems that deliver value.
🎯 Ready to make the leap?
💡 Apply for our internship and build your LLM Architecture portfolio today.