LLM Engineer's Career Bridge for Future in AI
The world of artificial intelligence is being reshaped by large language models (LLMs). Behind these intelligent systems are the LLM Engineers, a new breed of builders and enablers. If you’re looking to become one or are simply exploring what it takes—this is your career bridge into the future.
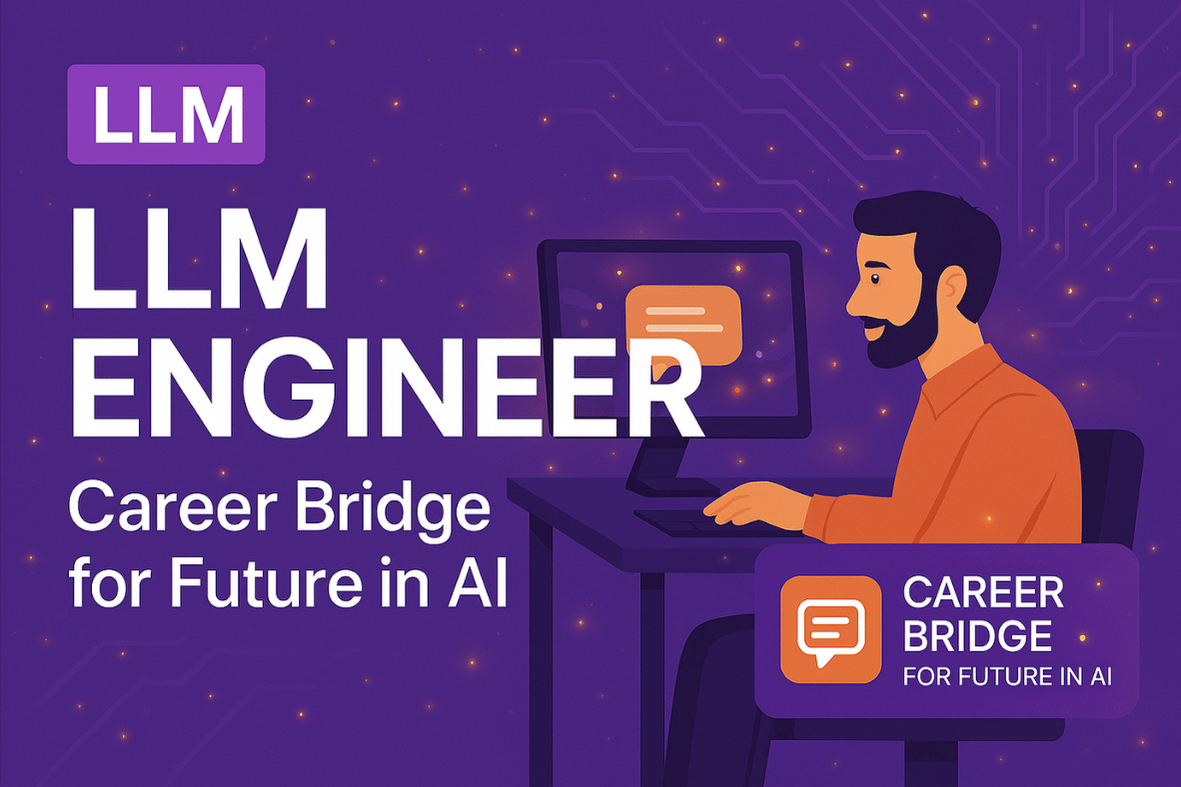
A Successful LLM Engineer
A successful LLM Engineer is a hybrid of software engineer, ML researcher, and systems thinker. They don’t just fine-tune models—they understand the trade-offs between different architectures, optimize for latency and scale, and build applications that harness the true potential of language models.
They can take an open-source base model or proprietary API and craft magical user experiences—chatbots, copilots, semantic search engines, and autonomous agents.
What You Will Learn
This guide is your launchpad. You'll learn:
- What defines an LLM Engineer in 2025 and beyond
- Key tools, frameworks, and capabilities to master
- How to prep for an LLM engineering interview (in 1 hour to 4 weeks)
- What recruiters look for in your resume
- How to gain experience—even if you're starting from scratch
Why This Role Matters in the AI Future
LLMs are at the core of next-gen AI systems. They enable:
- Human-like conversations in virtual assistants
- Knowledge workers to become 10x more productive
- AI agents to reason, plan, and act
- Enterprises to automate customer support, legal document analysis, code generation, and more
LLM Engineers are the ones who make this real—by understanding tokenization to prompt engineering, from fine-tuning to scalable deployment. As companies race to adopt AI-native architecture, LLM engineering is fast becoming one of the most in-demand skillsets in tech.
Key Success Factors for LLM Engineers
✅ Deep understanding of Transformer-based architectures (e.g., BERT, GPT, T5, LLaMA)
✅ Familiarity with prompt engineering, retrieval-augmented generation (RAG), and LoRA fine-tuning
✅ Strong programming skills (Python, PyTorch, Hugging Face)
✅ Ability to optimize models for latency, cost, and accuracy
✅ Knowledge of MLOps practices for LLMs: model versioning, deployment, monitoring
✅ Creativity to experiment with novel use cases and frameworks
Key Responsibilities of an LLM Engineer
- Fine-tune and optimize large language models for specific business tasks
- Design and develop RAG pipelines using vector databases (e.g., FAISS, Weaviate)
- Build APIs and microservices to serve LLM-based features
- Collaborate with prompt engineers, data scientists, and product managers
- Evaluate model performance through benchmarks, human feedback, and A/B testing
- Implement safeguards for bias, hallucination, and misuse
- Continuously monitor and retrain models based on user feedback and drift
Capabilities Needed for the Job
- Languages: Python (must), TypeScript (nice-to-have)
- Frameworks: Hugging Face Transformers, LangChain, OpenAI SDK, PyTorch
- LLM Ops: Weights & Biases, BentoML, Ray Serve, MLflow
- Data: Tokenization, embeddings, vector search, prompt templating
- Cloud Platforms: Azure OpenAI, AWS Bedrock, GCP Vertex AI
- Model Deployment: FastAPI, Docker, Kubernetes, serverless (e.g., Lambda)
- Security: Role-based access, rate limiting, red teaming for safety
- Model Evaluation: BLEU, ROUGE, perplexity, hallucination detection, truthfulness metrics
Top Tools to Learn and Crack the Interview
🛠️ LLM-Specific Tools:
- Hugging Face Transformers
- LangChain or LlamaIndex
- OpenAI or Anthropic APIs
- Ollama / LM Studio (for local testing)
- Vector databases (FAISS, Chroma, Pinecone)
- Prompt Engineering playgrounds (Flowise, Promptfoo)
📦 DevOps & Scaling Tools:
- Docker + Kubernetes
- Ray Serve or BentoML
- FastAPI + Redis queues
- Vercel or AWS Lambda for quick deployment
📚 Practice Resources:
- PapersWithCode (search: RAG, LoRA, alignment)
- LeetCode ML section + HF course
- Cohere or OpenAI cookbook repos
Top Keywords to Include in Your CV
- Large Language Model Engineering
- LLM Fine-tuning (LoRA, PEFT, RLHF)
- Prompt Engineering and Optimization
- Retrieval-Augmented Generation (RAG) Pipelines
- Vector Database Integration (FAISS, Pinecone)
- Scalable LLM Deployment (Docker, Kubernetes, Ray Serve)
- Model Monitoring and Drift Detection
- API Development with FastAPI / Flask
- MLOps for Generative AI
- OpenAI / Hugging Face Transformers Projects
Pro tip: Highlight results. Example → “Built RAG pipeline that reduced customer support response time by 35%.”
Don’t Have the Experience? Do Internship With Us.
🧠 Looking to break into LLM engineering but haven’t had hands-on opportunities yet?
Apply for our LLM Internship Program where you’ll:
- Fine-tune open-source models
- Build real-world generative AI applications
- Collaborate with mentors & contribute to GitHub repos
👉 Join us and build your LLM career from Day 1.
If You Only Had 1 Hour to Prepare for an LLM Engineer Interview
🔍 Focus your energy here:
- Understand prompt engineering: few-shot, chain-of-thought, system prompts
- Revise one LLM architecture (e.g., GPT-3.5, Mistral) and how attention works
- Be ready to explain your project—how you used, fine-tuned, or deployed an LLM
- Know how RAG works with vector DBs and a simple diagram
- Review one bug-fix or optimization you did in an LLM project
Prepare for It: 1 Day, 1 Week, 4 Weeks
✅ 1 Day Plan
- Review 2-3 key projects (LLM/RAG/fine-tuning)
- Watch a fast-paced YouTube crash course on LangChain or OpenAI
- Revise basics of tokenization, attention, inference APIs
- Practice 2 interview questions (system design + model debugging)
✅ 1 Week Plan
- Clone 1 open-source LLM project from Hugging Face or LangChain
- Implement a RAG system with ChromaDB or FAISS
- Learn and deploy a fine-tuned model using LoRA
- Document your work and add it to GitHub
- Practice with 3 mock interviews (technical + behavioral)
✅ 4 Week Mastery
- Complete 1 capstone project: Build an end-to-end app (e.g., Contract Summarizer, Code Explainer, AI Tutor)
- Read 2-3 seminal papers (e.g., “Attention is All You Need”, “InstructGPT”, “Retrieval-Augmented Generation”)
- Take a structured LLM course (e.g., Deeplearning.ai's Generative AI with LLMs)
- Create a portfolio with live demos, architecture diagrams, and GitHub links
- Mock interview with peers weekly, including whiteboard sessions
The world is shifting. AI isn't a buzzword anymore—it's the operating system of the future.
As an LLM Engineer, you’ll help write that future—one token at a time.
So whether you're just starting out or ramping up, this is your career bridge. Start building today.